The Bixbio Genomic Dataset
As part of the Illumina Accelerator program for startups in late 2021, Bixbio collected and sequenced the genomes of 750 Southern African individuals over the course of a few weeks. Just over half of this data forms the Bixbio South African Reference dataset: 397 whole-genome samples from healthy individuals with African or admixed-African ancestry, across 8 South African ethno-linguistic groups. It is to our knowledge the largest genomic dataset from Southern Africa.
This reference data has been at the core of Bixbio’s research and development since its creation, both internally as we work to unlock the power of diverse genomic data, and externally in support of local research efforts. The bioinformatics landscape has changed significantly since our first analysis of this dataset, however, and in bringing the reference data up to date Bixbio has recently had the pleasure of collaborating with the DRAGEN team at Illumina, who supported the reanalysis of the 312 highest-coverage samples by providing access to the latest version of the complete DRAGEN and PopGen pipelines [1]. Not only have variants of all sizes been called using state-of-the-art techniques – including multi-ethnicity reference genomes designed to reduce bias at the alignment stage of the analysis – but the Bixbio cohort has also been joint-genotyped with the entire 1000 Genomes Project (1KGP) dataset, effectively augmenting the latter with data from Southern African subpopulations (a region which was otherwise unrepresented).
Together the combined dataset provides a uniquely powerful resource for genomic research in Africa, the most genetically diverse region in the world. It includes 2,816 whole-genome samples, of which 973 are African; and 34 ethnicities, including 15 of recent African descent. A more in-depth look at the diversity of the dataset is presented elsewhere, including illustrative statistics related to genetic diversity at both the allelic and haplotype levels. We reproduce here, in Figure 1, a view of the distinct haplotypes observed in the region of the CYP2D6 gene as they occur in samples from different ethnicities, demonstrating one way in which global genetic diversity can to a large extent be viewed as a subset of African diversity.
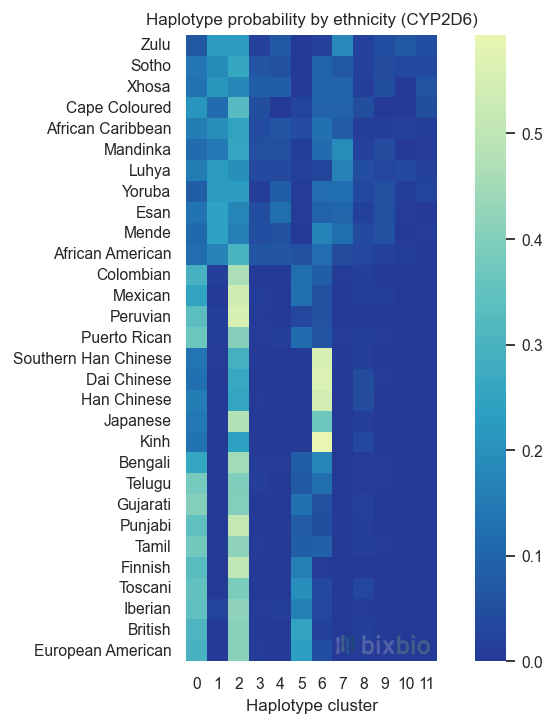
Perspective
The roughly 400 samples present in the Bixbio reference dataset may not seem like much compared to the scope of modern biobanks: the largest repository of genomic data in the world, for instance, is the UK Biobank, which currently consists of around 500,000 whole-genome samples; and the largest resource for aggregate genomic data, gnomAD, includes allele statistics from roughly 76,000 whole genomes. The UK Biobank is primarily made up of data from white individuals – approximately 95.6% of the dataset – alongside a small fraction of black individuals – roughly 1.6% [2,3]. (Recent UK census data reports values of 83.1% and 3.7% for these ethnic groups respectively, for comparison [4,5].) On the other hand, gnomAD’s whole-genome dataset is significantly more diverse: although 51.7% of its samples are from European ethnicities (not necessarily white), 27.2% are from African or admixed African groups [6].
What does this mean in terms of sample numbers? Well, combined, two of the largest genomic datasets in the world include 28,926 (8,182 + 20,744) whole-genome samples from individuals of African descent. In addition to the fact that this is an alarmingly small number, there are three things to note here:
Almost no genomic data comes from Southern Africa – let alone South Africa. Due to the fact that very little genetic sequencing occurs on the African continent (a disparity that is unfortunately growing [7]), much of the African-ancestry data that does exist represents African American, West African, and East African subpopulations; primarily from more developed African nations such as Nigeria and Kenya. This is a crucial omission, not just because there is more genetic diversity within the African continent than there is outside of it (see again our related article), but because these datasets are used to improve both bioinformatics and medical research and methods – and research has shown that the under-representation of ethnic groups can lead to inequalities in the efficacy of such methods [8].
All genomic data is not created equal, because large data sets are analysed using a variety of software pipelines. The sequencing and analysis of genomic data – the ‘calling’ of variant alleles from DNA ‘reads’ – is a complex, constantly evolving science. The last few years have seen, for instance, the introduction of long-read sequencing [9], machine learning-based variant-calling algorithms [10], and non-linear reference ‘pangenomes’ [11]. While this is in general a good thing for diverse data sets, it introduces real complications, since the outputs of different pipelines – and often even versions of the same pipeline – can generally not be compared or combined directly. The UK Biobank’s whole-genome data, for example, was analysed using two different pipelines, the most recent of which was released in 2020 and has been superseded by multiple newer versions. The gnomAD dataset is far more complex, involving data from more than 300 sources, and as such its maintainers were required to develop a pipeline of their own to combine and standardise disparate data [12]. The point here is that if the goal is a statistical analysis of a large number of genomes, the analysis procedure matters arguably as much as the composition of the dataset; and it isn’t good enough for African genomic data to simply exist: it needs to be analysed correctly as well.
Not all genomic data is equally accessible. This is perhaps perfectly understandable when one considers what whole-genome data is: a complete record of an individual’s DNA sequence. The ethical concerns surrounding the capture, access, and study of any genomic data are not trivial – especially when it comes to under-represented and previously disadvantaged people groups. The UK Biobank data contains individual whole-genome data, and researchers who apply for access to the data undergo a comprehensive vetting procedure. The gnomAD data is aggregated, i.e., each variant allele in the database is annotated with the frequency with which it occurs in certain subpopulations – but it is impossible to tell which alleles any single individual has. The gnomAD data is publicly accessible for this very reason: that genomes cannot be directly accessed.
There are two important, diverse, publicly accessible datasets that are exceptions to some or all of the above points: the 1000 Genomes Project (1KGP [13]) and the Human Genome Diversity Project (HGDP [14]). Both of these datasets include significant numbers of samples from globally diverse ethnicities, and both are publicly accessible. The 1KGP dataset, too, is consistently reanalysed using up-to-date pipelines (by, for instance, Illumina [15]) to ensure that it remains a valuable resource for global genomic research. The 1KGP cohort consists of 2,504 samples (excluding related individuals), of which 661 are of African ancestry (including 157 African American and African Caribbean samples). The HGDP cohort consists of 828 samples, of which 88 are from Africa (6 from Southern Africa). Both of these datasets are included in and contribute to gnomAD’s statistics.
The Bixbio dataset
In this light, the reanalysis of the Bixbio reference dataset in concert with the 1KGP cohort is perhaps more significant: not only does it significantly bolster the number of African genomes available to researchers such as ourselves, but it also provides an unprecedented view of Southern African genetics using the most advanced analysis pipelines possible. Figures 2 and 3 provide a simple overview of the African ethnicities included in the dataset.
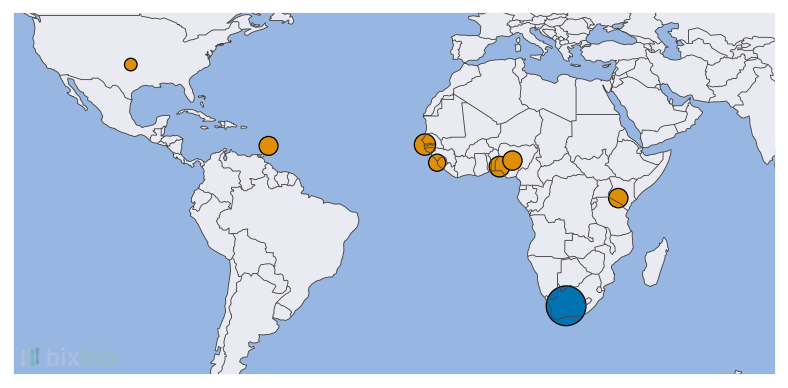
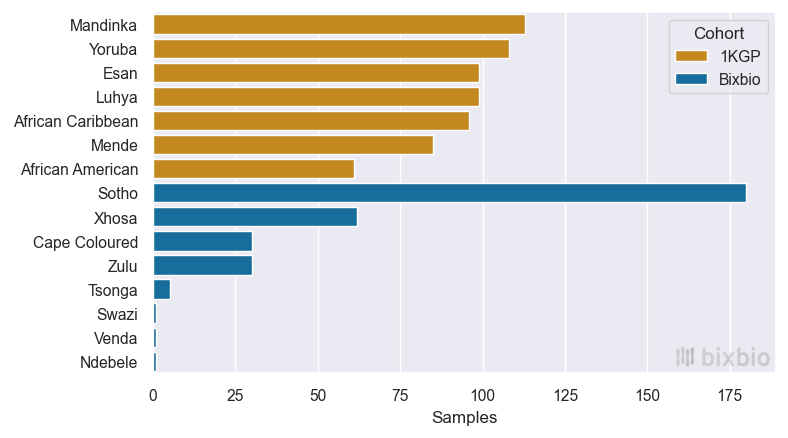
The size and diversity of the Bixbio dataset should perhaps give one pause: not because of how much additional data it provides (it nearly matches the number of African samples and ethnicities in the 1KGP cohort), but because of what it still fails to capture. South Africa is one of the most diverse countries in the world – it is home to significant subpopulations of African, Indian, white, and coloured1 ethnicities, and has 11 official spoken languages. While Bixbio’s dataset compares favourably to both the 1KGP and HGDP cohorts in terms of both sample and ethnicity numbers, it doesn’t yet represent the full scope of genetic diversity present in South Africa. And if there remains work to be done in this regard within a single Southern African country, how much more is required before we have access to a truly African (and a truly global) genomic dataset?
That is, of course, a long-term goal. For now, the Bixbio cohort has already proved indispensable to research projects at Bixbio, and is now more useful than ever thanks to this reanalysis. As a company and as a team we are excited, and continually encouraged, to play a small part in advancing genomic analysis from our unique, Southern African perspective. The goal, as always, is to create solutions that focus on what makes diverse data so powerful, and to use these approaches to transform genomic medicine for everyone.
Data access
The Bixbio South African Reference dataset was created to serve as a reference for genetic variation in Southern Africa and beyond, and can in specific circumstances be made available to researchers working on projects that have the potential to positively impact the communities involved (e.g., genetic research related to locally prevalent medical conditions, or the development of tools to improve the accuracy of bioinformatics in Africa). Interested parties should contact the Bixbio team directly.
Acknowledgments
Bixbio has been fortunate enough to work with many individuals who are similarly passionate about advancing diversity in genomics. We are extremely to grateful to Illumina, and in particular the DRAGEN team, for their support in creating the dataset described here. The sequencing of the Bixbio samples was made possible thanks to the Illumina Accelerator program in Cambridge, UK, and this combined reanalysis of the 1KGP/Bixbio dataset was again enabled by members of the DRAGEN team. We thank Ursula Arndt2, Shyamal Mehtalia, and Rami Mehio in particular.